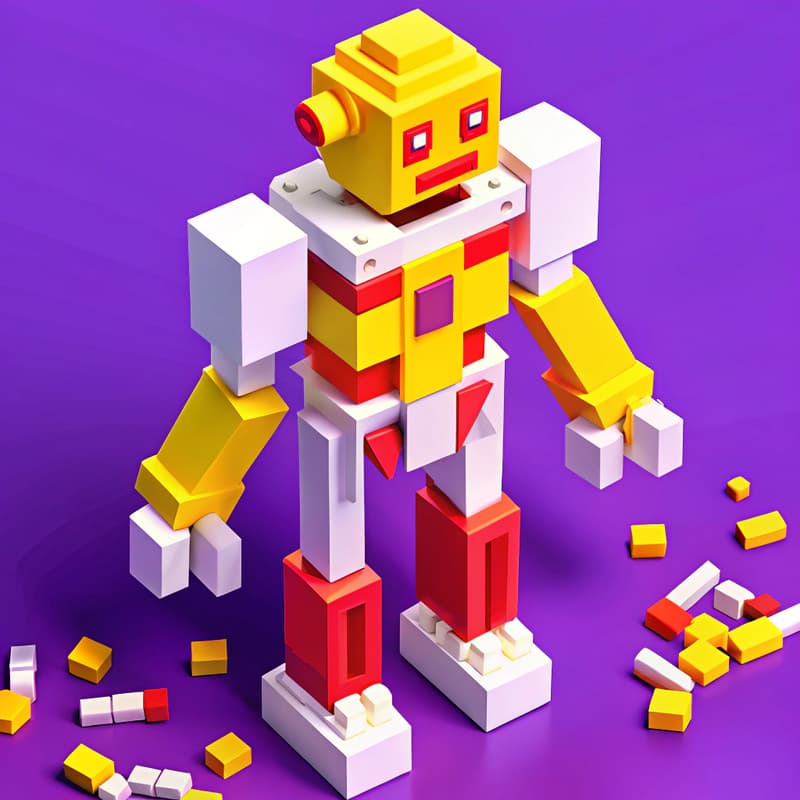
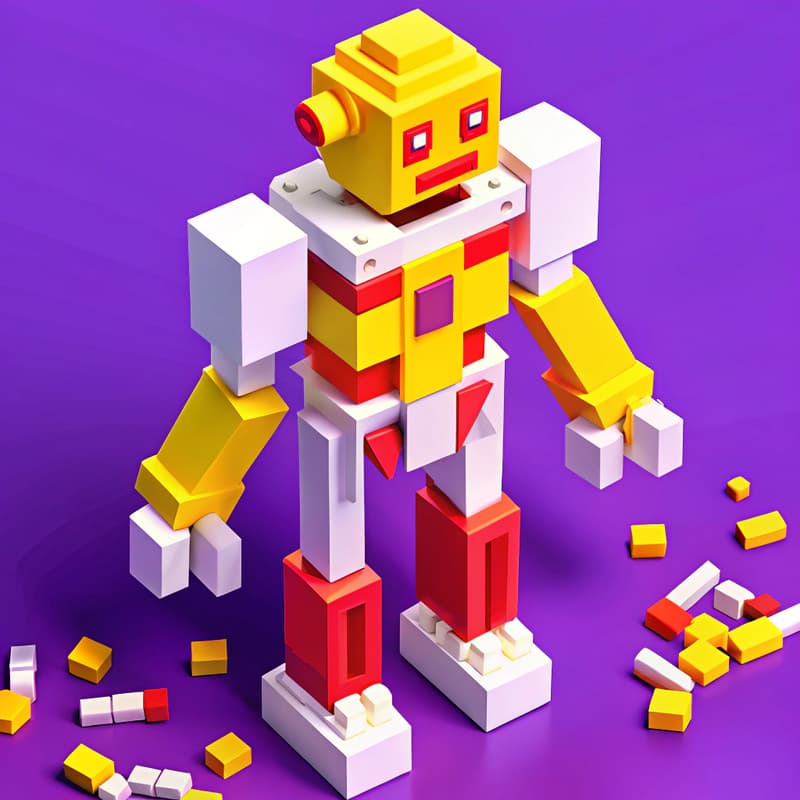

AI Risk 101: How to test your AI systems before users do
You’ve built an AI system. It works in demos. But can you really trust it in the wild?
AI breaks in ways regular software doesn’t. It can hallucinate, say risky things, leak data, or perform inconsistently with unexpected inputs. These aren’t just engineering bugs — they’re product risks: brand damage, compliance violations, lost trust.
To build AI apps that are reliable, safe, and production-ready, you need a systematic, repeatable AI testing process that aligns with your use case, industry, and internal policies.
Join this hands-on webinar to learn how to identify AI risks, define test strategies, and introduce structured testing into your AI product workflow.
We’ll cover:
⚠️ Common AI failure modes: hallucinations, unsafe outputs, jailbreaks, PII leaks, brand risks
✅ Testing techniques — stress tests, red-teaming, regression testing for LLMs
🔡 How to create and use synthetic data for AI testing
💻 Practical example: testing AI app with Evidently
Who is it for?
This session is for AI product teams, AI risk and governance experts, ML engineers, data scientists, and tech leads who are:
Building or deploying LLM-powered applications
Concerned about real-world risks like unsafe outputs and compliance issues
Looking to move beyond "vibes-based" testing and towards structured, policy-aligned evaluation workflows
Speaker:
Elena Samuylova — CEO & Co-founder at Evidently AI, the company behind Evidently, an open-source framework for AI evaluation with 25+ million downloads.
