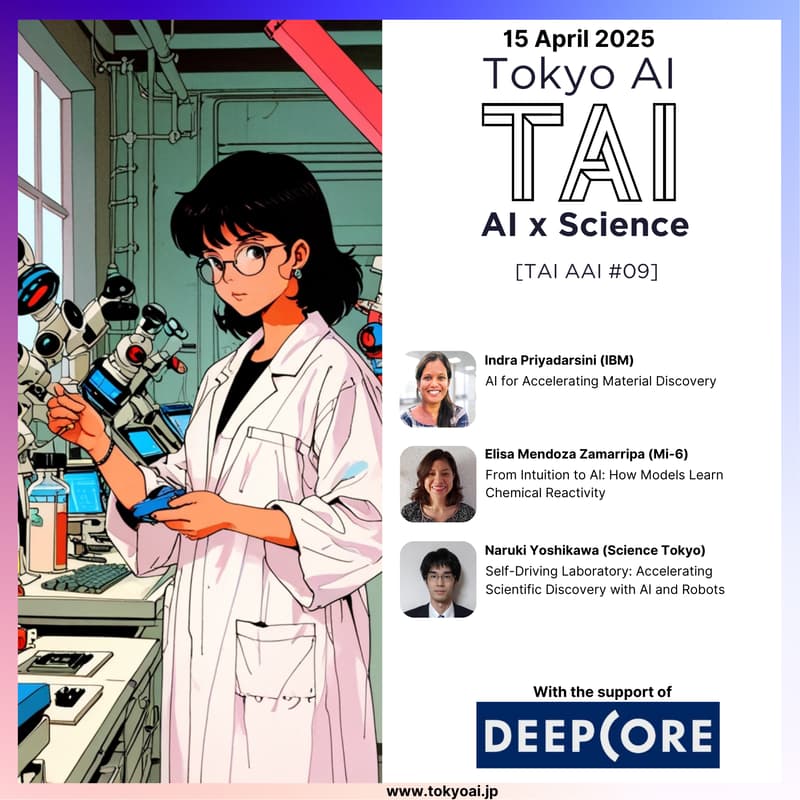
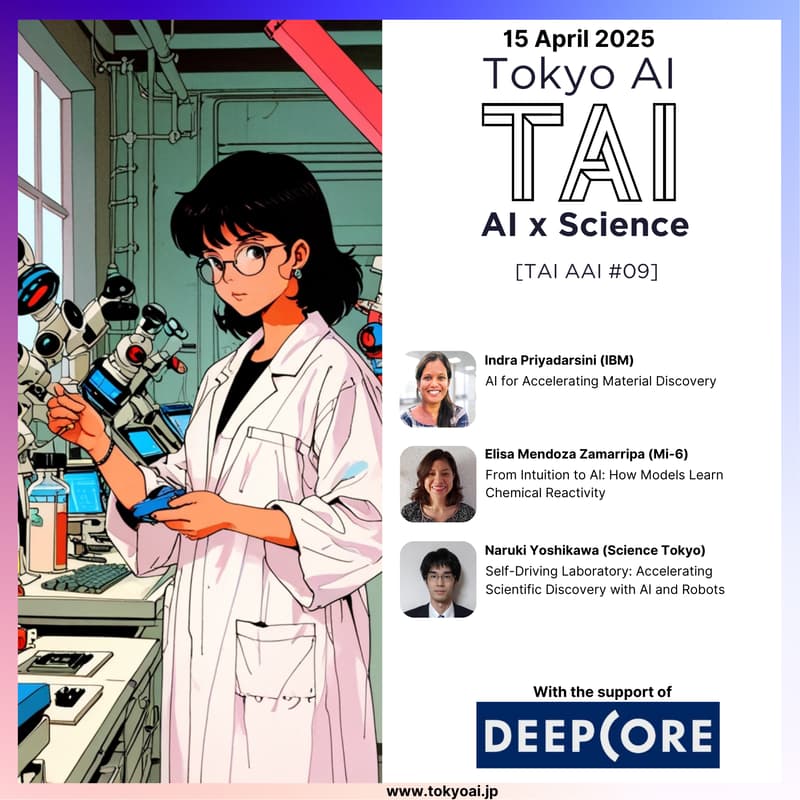

TAI AAI #09 - AI x Science
This Tokyo AI (TAI) Advanced AI (AAI) group session on AI in science and scientific discovery.
Schedule
18:30 - 19:00 Doors open
19:00 - 19:10 Introduction
19:10 - 19:40 AI for Accelerating Material Discovery (Indra Priyadarsini)
19:40 - 20:10 From Intuition to AI: How Models Learn Chemical Reactivity (Elisa Mendoza Zamarripa)
20:10 - 20:40 Self-Driving Laboratory: Accelerating Scientific Discovery with AI and Robots (Naruki Yoshikawa)
20:40 - 21:30 Networking
Speakers
Indra Priyadarsini (profile)
Title: AI for Accelerating Material Discovery
Abstract: Foundation models, pre-trained on vast and diverse datasets, have revolutionized various domains of artificial intelligence, notably in natural language processing and computer vision. In this talk, we explore the emerging role of foundation models in the fields of materials science and chemistry. We will discuss how these models can be leveraged to accelerate the discovery of new materials, predict molecular properties, and enhance our understanding of complex chemical processes. By integrating foundation models into research workflows, researchers can achieve new levels of precision and efficiency, paving the way for breakthroughs that were previously beyond reach. The talk will also address current limitations and future directions, emphasizing the need for continued innovation to fully harness the power of these models.
Bio: Indra Priyadarsini received her Ph.D. from Shizuoka University in 2022, where she specialized in optimization algorithms and deep learning. She is currently a Research Scientist at IBM Research – Tokyo, focusing on models and algorithms for multimodal foundation models in material discovery. Her work advances AI-driven approaches for accelerated scientific discovery. She is a recipient of the IPSJ Industrial Achievement Award 2024 for her contributions towards AI-based material discovery.
Elisa Mendoza Zamarripa (profile)
Title: From Intuition to AI: How Models Learn Chemical Reactivity
Abstract: Chemistry often relies on the experience and intuition of chemists to understand molecular behavior—knowing which molecules are likely to react, and under what conditions. But what happens when we ask machines to do the same? In this presentation, we will explore how artificial intelligence (AI) models learn chemical reactivity and predict chemical reactions—what will react, where, and how successfully. We will focus on advanced machine learning techniques like Graph Neural Networks (GNNs) that “read” molecules as graphs, and Large Language Models (LLMs) that identify chemical patterns from vast amounts of textual data. While these methods promise to accelerate fields like drug discovery and materials development, they also bring up important questions: How do these models actually learn chemistry? Can they explain their predictions? And how much should we rely on them versus human expertise? This talk aims to open a discussion about how AI systems can not only predict chemistry but also help us understand it.
Bio: Elisa has a PhD in Biomedical Engineering from Tokyo Tech (now Institute of Science Tokyo), specializing in biomaterials and molecular interactions at biointerfaces. After graduating, she joined MI-6 Ltd. as a data scientist. She has worked on building tools for the prediction of material properties and screening applications. She also has international experience in biomedical engineering, having worked in Mexico and The Netherlands.
Naruki Yoshikawa (profile)
Title: Self-Driving Laboratory: Accelerating Scientific Discovery with AI and Robots
Abstract: Artificial intelligence is transforming a wide range of fields, and scientific research is no exception. One promising advancement is the development of self-driving laboratories, automated research environments where AI-powered robots conduct scientific experiments autonomously. In this talk, I will explore the current state of self-driving laboratory technology and discuss my own work focused on automating experiments using robot arms, as well as developing natural language interfaces for controlling these robots.
Bio: Naruki Yoshikawa is an assistant professor at the Institute of Science Tokyo. He obtained his Ph.D. in 2024 from the Department of Computer Science at the University of Toronto, where he studied the automation of chemistry experiments. He received his master’s degree in 2020 and his bachelor’s degree in 2018, both from The University of Tokyo.
Our Community
Tokyo AI (TAI) is a community composed of people based in Tokyo and working with, studying, or investing in AI. We are engineers, product managers, entrepreneurs, academics, and investors intending to build a strong “AI coreˮ in Tokyo. Find more in our overview: https://bit.ly/tai_overview
Organizers
Kai Arulkumaran: Research Team Lead at Araya, working on brain-controlled robots as part of the JST Moonshot R&D program. Previously, he completed his PhD in Bioengineering at Imperial College London and had work experience at DeepMind, FAIR, Microsoft Research, Twitter Cortex, and NNAISENSE. His research areas are deep learning, reinforcement learning, evolutionary computation, and computational neuroscience.
Gergely (Greg) Juhasz: Associate Professor at the Institute of Science Tokyo (formerly Tokyo Tech). His expertise lies in computational chemistry, and he is a member of the TAC-MI program, which bridges Chemical and Materials Science with Data Science and Machine Learning. He earned his PhD in Materials Chemistry at Kyushu University and has research experience at Carnegie Mellon University, Kyushu University, and Tokyo Tech. His research focuses on quantum chemical and computational approaches to problems in nanocarbons, catalysis, and electrochemical systems.
Ilya Kulyatin: Fintech and AI entrepreneur with work and academic experience in the US, Netherlands, Singapore, UK, and Japan, with an MSc in Machine Learning from UCL.
Sponsor
DEEPCORE is a Tokyo-based AI-focused incubator and VC firm supporting AI Salon Tokyo.