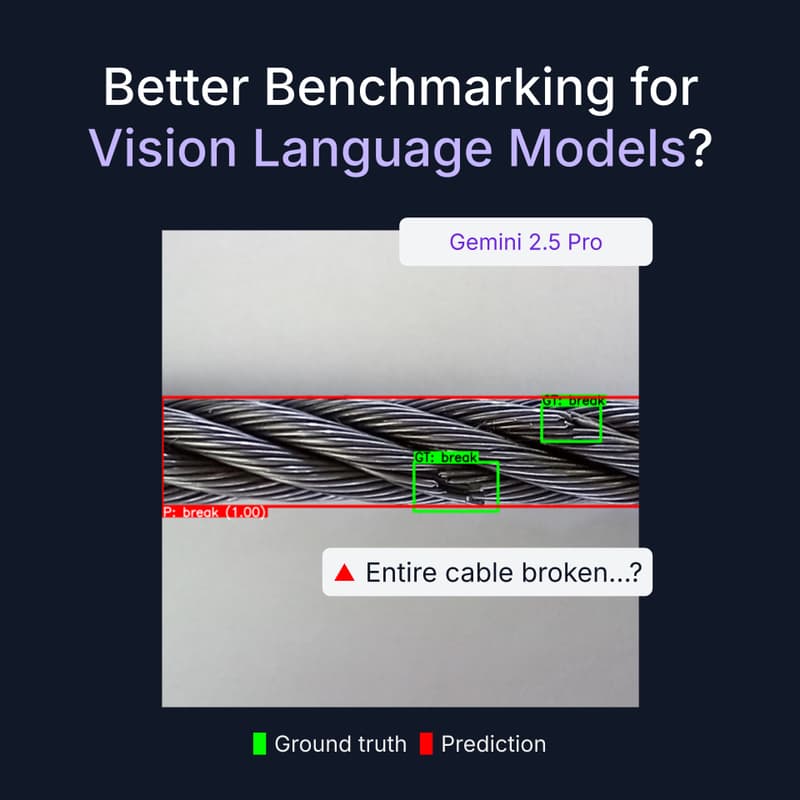
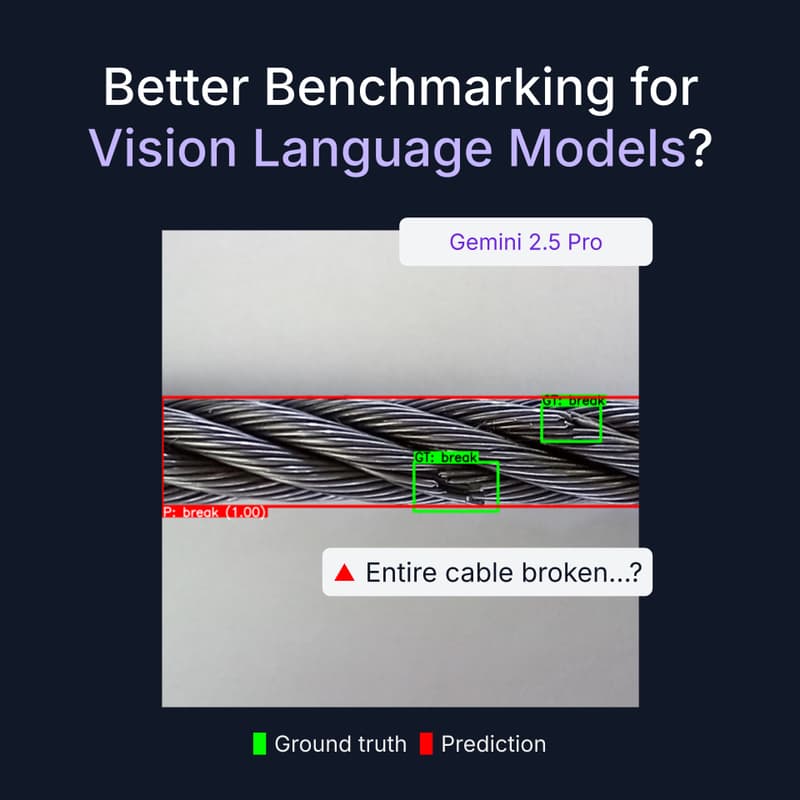
Better Benchmarking for AI Object Detection Models
How do large vision-language models (VLMs) perform on less common objects? In this session, we will examine situations where larger VLMs tend to fail at object detection and explore when purpose-built detection models perform better.
Vision-language models (VLMs) trained on internet-scale datasets achieve impressive zero-shot detection performance on common objects. However, VLMs can struggle to generalize to less common, "out-of-distribution" classes, tasks, and imaging modalities not typically found in their pre-training. This directly limits their impact in real-world applications.
Rather than simply re-training VLMs on more visual data to solve this issue, we will explore how VLMs can be aligned with annotation instructions containing a few visual examples and rich textual descriptions. To illustrate this, we will introduce Roboflow’s RF100-VL, a large-scale collection of 100 multi-modal object detection datasets with diverse concepts not commonly found in VLM pre-training.
We will discuss our evaluation of state-of-the-art models on our benchmark in zero-shot, few-shot, semi-supervised, and fully-supervised settings, providing a comprehensive overview of the current state of VLMs and specialized object detection models for object detection.
Every Thursday at 11am ET we will showcase an exciting new feature or product. This webinar is open to everyone, we hope to see you there!
We'll also make time at the end for open Q&A.