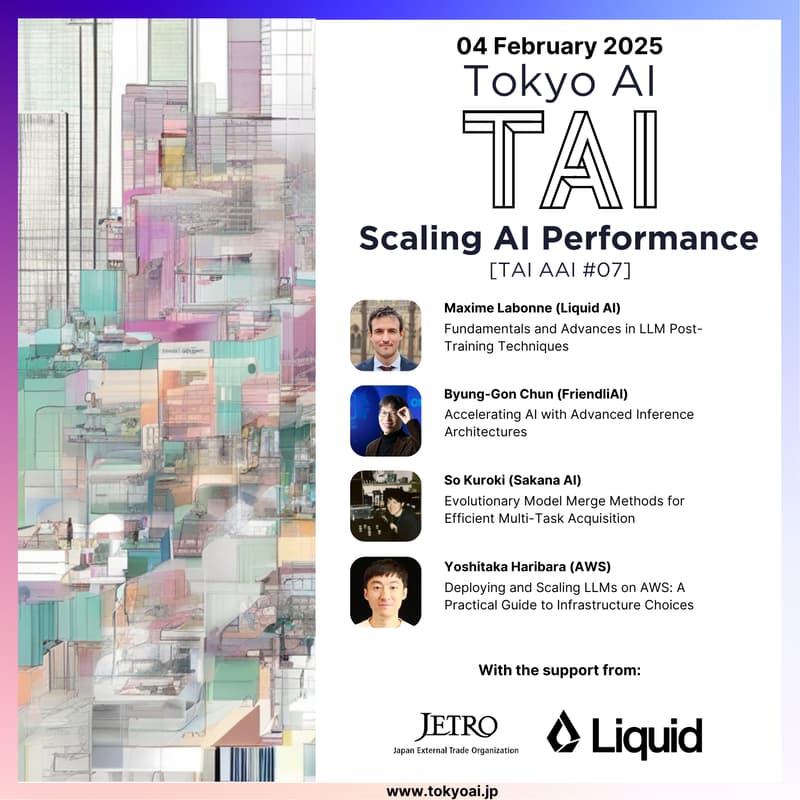
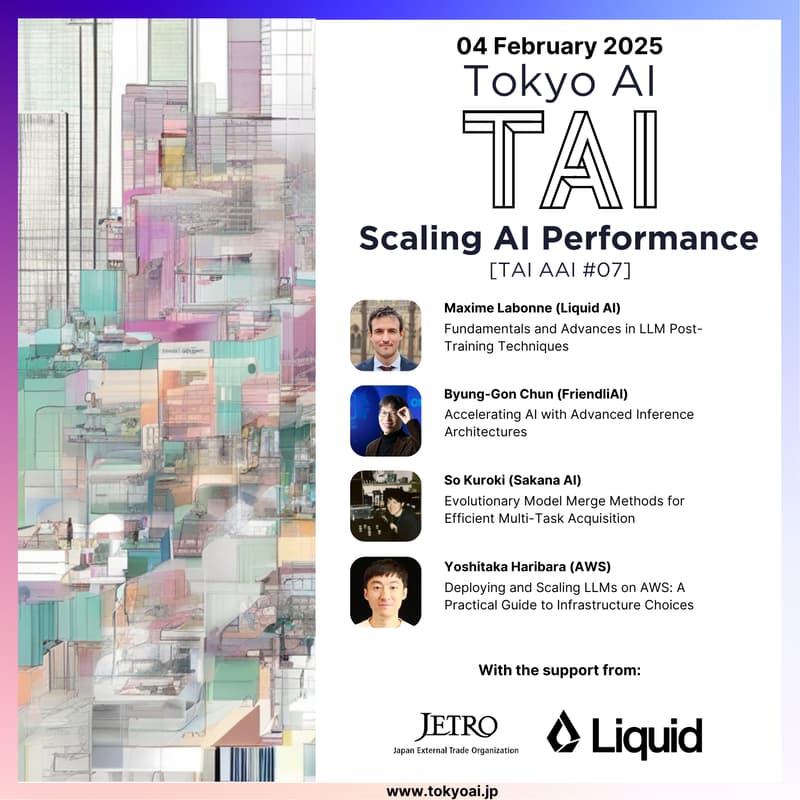
TAI AAI #07 - Scaling AI Performance
Note: we often vet the participants based on their background and fit to the sessions (just "interest" is often not enough, to assure the network value is maximized). Please don't take a rejection personally and try join us again!
This session of the Tokyo AI (TAI) Advanced AI (AAI) group features technical speakers from leading global teams at the forefront of large model training, inference engineering, and research. We're excited to welcome two overseas guests: Maxime Labonne from Liquid AI (visiting from London) and Byung-Gon Chun from FriendliAI (Redwood City/US and Seoul).
Liquid AI, an MIT-originated startup co-founded by Daniela Rus (Director of MIT CSAIL) and Ramin Hasani (an MIT AI rising star), recently raised $250M and is expanding its presence in Tokyo.
FriendliAI, a US-based startup (Redwood City, CA), is making waves with its GPU-optimized hardware stack, achieving record-breaking inference speeds (now also available via HuggingFace).
Rounding out the evening, we’ll hear from our local heroes at Sakana AI, presenting their groundbreaking research on evolutionary model merging, a paper just accepted at ICLR '25.
The speakers will share their unique expertise on:
Post-training techniques to fine-tune large language models for real-world applications.
Optimization strategies to accelerate generative AI inference using GPU-optimized architectures.
Evolutionary approaches to model merging for efficient multi-task acquisition in the open-source era.
Schedule
17:30 - 18:00 Doors Open
18:00 - 18:05 Introduction
18:05 - 18:35 Fundamentals and Advances in LLM Post-Training Techniques (Maxime Labonne, Liquid AI)
18:40 - 19:10 Accelerating AI with Advanced Inference Architectures (Byung-Gon Chun, FriendliAI)
19:15 - 19:45 Evolutionary Model Merge Methods for Efficient Multi-Task Acquisition (So Kuroki, Sakana AI)
19:45 - 20:10 Deploying and Scaling LLMs on AWS: A Practical Guide to Infrastructure Choices (Yoshitaka Haribara, AWS)
Our Community
Tokyo AI (TAI) is a community composed of people based in Tokyo and working with, studying, or investing in AI. We are engineers, product managers, entrepreneurs, academics, and investors intending to build a strong “AI coreˮ in Tokyo. Find more in our overview: https://bit.ly/tai_overview
Speakers
1. Maxime Labonne (Head of Post-training at Liquid AI)
Title: Fundamentals and Advances in LLM Post-Training Techniques
Abstract:
In this talk, we will discuss the fundamentals of modern LLM post-training with concrete examples. High-quality data generation is at the core of this process, and we will focus on the accuracy, diversity, and complexity of the training samples.
We will explore key training techniques, including supervised fine-tuning and preference alignment. Finally, we will focus on different approaches to evaluation, discussing their pros and cons for measuring model performance.
We will conclude with an overview of emerging trends in post-training methodologies and their implications for the future of LLM development.
Bio:
Maxime Labonne is Head of Post-Training at Liquid AI. He holds a Ph.D. in Machine Learning from the Polytechnic Institute of Paris and is a Google Developer Expert in AI/ML.
Maxime is a world-leading expert on post-training. He has made significant contributions to the open-source community. Maxime also gave a lot to the community, authoring the LLM Course, producing a large number of tutorials on fine-tuning, building tools such as LLM AutoEval, and several state-of-the-art models like NeuralDaredevil.
Additionally, Maxime is the author of the best-selling books “LLM Engineer’s Handbook” and “Hands-On Graph Neural Networks Using Python”.
2. Byung-Gon Chun (CEO, FriendliAI)
Title: Accelerating AI with Advanced Inference Architectures
Abstract:
The era of generative AI-based agentic AI is upon us. By applying more computations during inference, we are unlocking the full potential of AI capabilities. As these services scale, achieving fast and efficient inference becomes more critical than ever.
In this talk, I will explore optimization techniques for accelerating generative AI inference, supported by case studies demonstrating their effectiveness in specific scenarios. Building on these foundations, Gon will also introduce the Friendli Endpoints service, powered by Friendli Inference. This service outperforms alternative providers by using their proprietary GPU-optimized stack. According to Artificial Analysis, FriendliAI is the fastest GPU-based API provider.
Bio:
Byung-Gon Chun is the CEO and Co-founder at FriendliAI, a leading company in generative AI acceleration, and a professor in the CSE department at Seoul National University (SNU). He has published world-renowned research in AI, for instance on continuous batching.
Previously, he worked at Facebook, Microsoft, Yahoo!, and Intel. Gon holds a Ph.D. in CS from UC Berkeley, a M.S. in CS from Stanford University, and both a M.S. and a B.S. from SNU.
He has received the 2021 EuroSys Test of Time Award and the 2020 ACM SIGOPS Hall of Fame Award and has been honored with research awards from Google, Amazon, Facebook, and Microsoft.
3. So Kuroki (Research Engineer, Sakana AI)
Title: Evolutionary Model Merge Methods for Efficient Multi-Task Acquisition
How can we efficiently acquire a model that can solve several tasks? Model merging has emerged as a powerful strategy for consolidating specialized foundation models into a single model. This talk introduces two evolutionary approaches—Evolutionary Recipe Optimization and CycleQD.
Evolutionary Recipe Optimization focuses on evolving the “recipe” for combining model parameters, using evolutionary algorithms to explore how best to fuse fine-tuned models. In contrast, CycleQD uses Quality-Diversity principles to evolve the parameter space itself, optimizing each task’s performance while retaining diverse, specialized skills.
We will discuss the design considerations behind both methods, their empirical performance on diverse tasks, and the broader implications for efficient, scalable multi-task model merging in the open-source era.
Bio:
So Kuroki is a research engineer at Sakana AI, focusing on developing LLM agents and enhancing their skill acquisition. His research interests and experiences align with collective intelligence (learning and robotics) and AI collaboration with humans (HCI). He loves to see agents become smarter by interacting and sharing their knowledge, and likewise, he loves to see AI empower humans through collaboration.
Before joining Sakana AI, he worked in robot learning at OMRON SINIC X and in Yutaka Matsuo’s Lab at The University of Tokyo. He holds a bachelor’s and a master’s degree from the University of Tokyo.
4. Yoshitaka Haribara (Sr. Solutions Architect at AWS)
Title: Deploying and Scaling LLMs on AWS: A Practical Guide to Infrastructure Choices
Abstract:
Foundation Models and LLMs now range from billions to trillions of parameters, presenting unique deployment challenges. Organizations must navigate tradeoffs between cost, operational efficiency, and implementation complexity.
This talk provides a practical guide to deploying LLMs on AWS and hardware accelerators, including GPU and AI chips (AWS Trainium and Inferentia). Yoshitaka will offer step-by-step guidance for deployment using real-world examples like DeepSeek-R1 and its Distill variants and best practices for optimizing cost and performance at scale.
Bio:
Yoshitaka Haribara is a Sr. GenAI/Quantum Startup Solutions Architect at AWS and Visiting Associate Professor at Center for Quantum Information and Quantum Biology (QIQB), The University of Osaka. At AWS, he works with leading Japanese generative AI startups including Sakana AI, ELYZA, and Preferred Networks (PFN). He supports Japanese model providers to develop Japanese LLMs and list them (PFN, Stockmark, and Karakuri LLMs) on Amazon Bedrock Marketplace. With his background in combinatorial optimization with quantum optical devices, Yoshitaka also guides customers in leveraging Amazon Braket for quantum applications and works to bring Japanese quantum hardware to AWS. He holds a Ph.D. in Mathematical Informatics from The University of Tokyo and a B.S. in Mathematics from The University of Osaka.