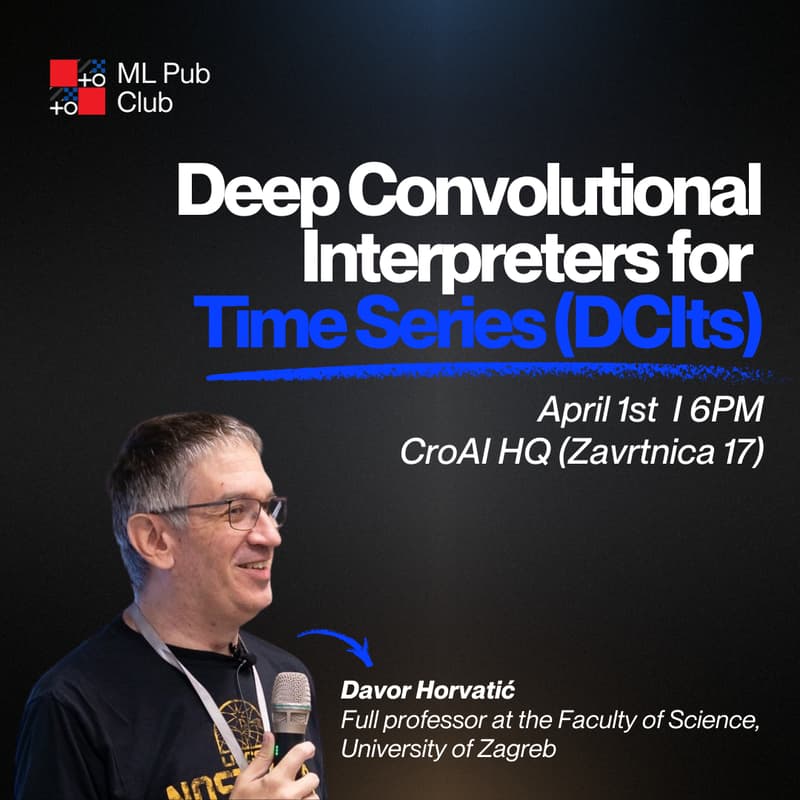
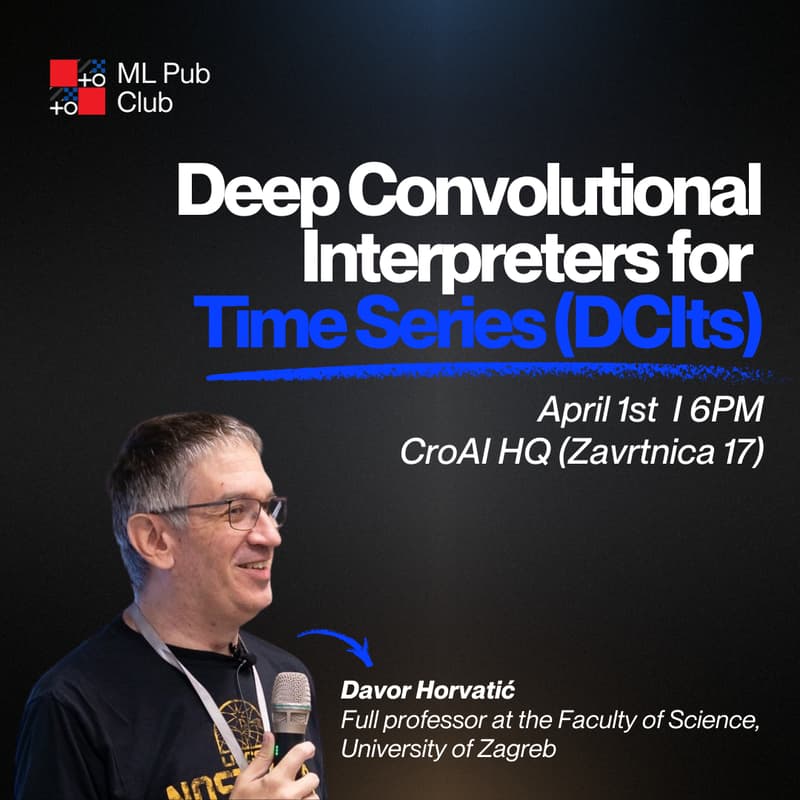
ML Pub Club #20: Deep Convolutional Interpreters for Time Series (DCIts)
A new DNN time series forecasting paradigm has emerged—one that excels in interpretability by unlocking the generating process behind each forecast.
Deep Convolutional Interpreters for Time Series (DCIts) delivers sample-specific insight by generating dynamic processes and identifying the most influential time series and their lagged interactions. DCIts offers local interpretability that traditional global regression models can't match. This innovation eliminates the need for computationally intensive per-sample regressions while adapting seamlessly to changing dynamics.
At the upcoming ML Pub Club, we will be joined by Professor Davor Horvatić, a full professor at the Faculty of Science, University of Zagreb. His research spans elementary particle physics and complex systems, strongly focusing on time series analysis and developing innovative AI models. With over 50 publications cited over three thousand times, his work has covered topics from quantum field theory at finite temperature and density to complexity measures in time series and their dynamical processes.
Davor will present research from the paper "DCIts – Deep Convolutional Interpreter for Time Series" (https://arxiv.org/abs/2501.04339) and guide us through the inner workings of this state-of-the-art model. Attendees will explore:
How DCIts provides transparent, per-sample explanations for forecasts.
The mechanisms that allow it to select optimal input windows and higher-order interactions automatically.
Why DCIts represents a deep neural network model with implicit local adaptation and inherent interpretability
See you April 1st, and no - it is not a joke! ;)